Edwin V. Bonilla
Senior Principal Research Scientist, CSIRO's Data61.
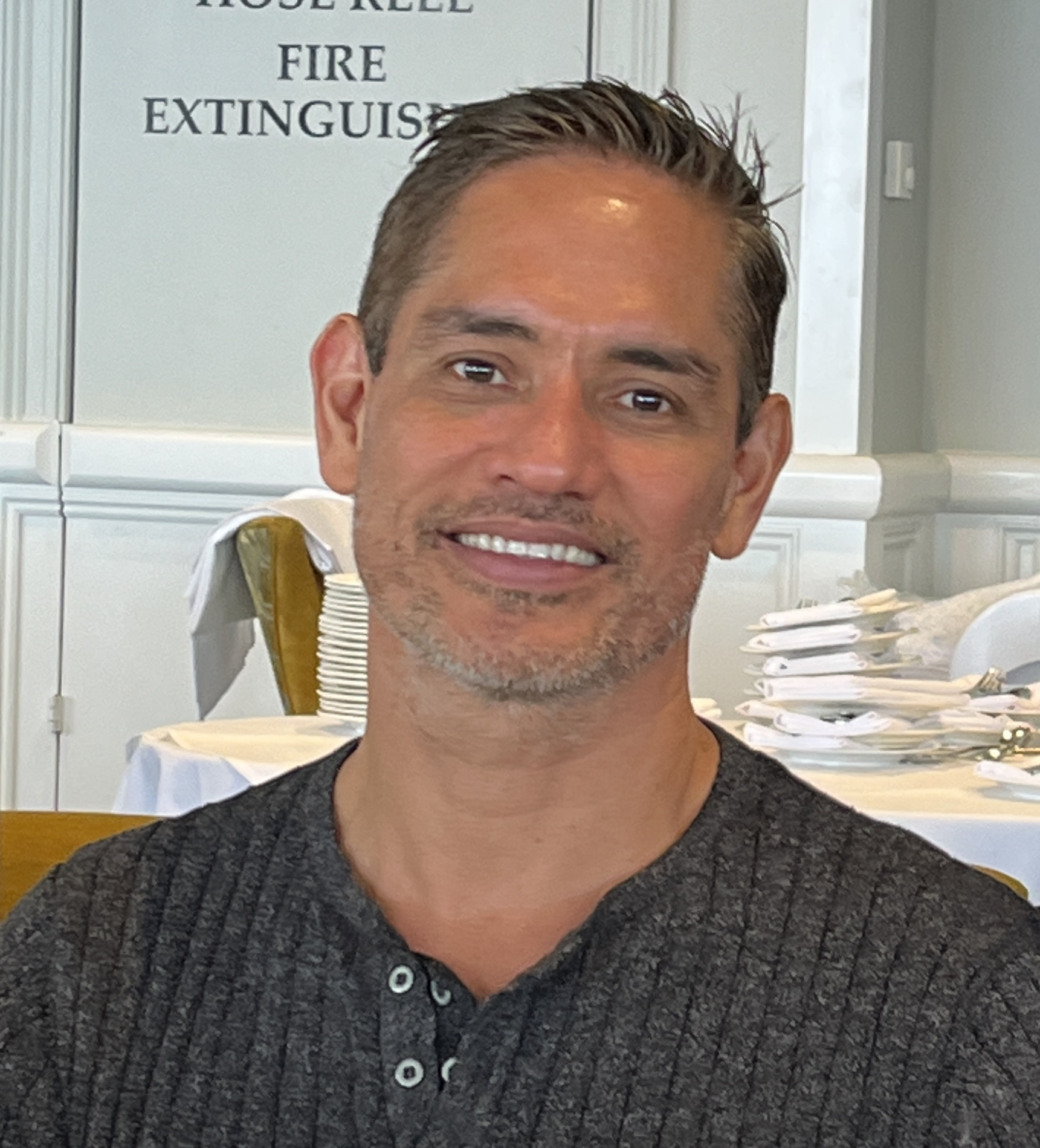
edwin.bonilla [at] data61.csiro.au
“It’s not [only] the consequence that makes a problem important, it is that you have a reasonable attack.”
Richard Hamming, 1986.
I am a senior principal research scientist and Science Leader for Foundational Machine Learning of the Analytics and Decision Sciences Research program at CSIRO’s Data61, Australia. I also holds an Honorary Associate Professor position at the Australian National University (ANU). Before joining CSIRO, I was a Senior Lecturer at the University of New South Wales (UNSW, 2014-2018), a Senior Researcher at National ICT Australia (NICTA, 2010-2014) and a Research Associate at The University of Edinburgh (UK, 2007-2009).
I obtained a PhD in Informatics at the University of Edinburgh in 2008 and a MSc in Artificial Intelligence with Distinction at the University of Edinburgh (2004). Most of my expertise is in probabilistic modelling and inference algorithms for the analysis of complex data, in areas such as scalable Bayesian inference, Gaussian processes and multi-task learning. I have worked in applications such as geophysical inversions, spatio-temporal modelling, computer vision and document analysis. My current interests include causal discovery and inference, Gaussian processes, Bayesian optimization, optimal design of experiments, neural differential equations and graph neural networks.
My research vision is that of making modern probabilistic machine learning a fundamental part of decision making. This vision is driven by challenging problems in causal discovery and inference and the optimal design of experiments, and underpinned by principled modelling, quantification and propagation of uncertainty. The output of my research is focused on top machine learning venues such as NeurIPS, ICML and AISTATS.
news
Jun 25, 2024 | DAG Estimation at ICML 2024 |
---|---|
Jun 25, 2024 | Contextual DAGs at AISTATS 2024 |
Aug 25, 2023 | Best student research paper award at KDD 2023 |
latest posts
highlighted publications
- Contextual directed acyclic graphsIn International Conference on Artificial Intelligence and Statistics (AISTATS) , 2024
- Variational DAG Estimation via State Augmentation With Stochastic PermutationsarXiv preprint arXiv:2402.02644, 2024
- Optimal Transport for Structure Learning Under Missing DataAccepted for publication at International Conference on Machine Learning (ICML), 2024
- ProDAG: Projection-induced variational inference for directed acyclic graphsarXiv preprint arXiv:2405.15167, 2024
- Parameter Estimation in DAGs from Incomplete Data via Optimal TransportIn Accepted for publication at International Conference on Machine Learning (ICML) , 2024